PLS regression is also used to build predictive models. The equation of the PLS regression model writes: Principal component analysis of a block A block is essentially unidimensional if the first eigenvalue of the correlation matrix of the block MVs is larger than 1 and the second one smaller than 1, or at least very far from the first one. Two other schemes for choosing the inner weights exist: The matrix B of the regression coefficients of Y on X, with h components generated by the PLS regression algorithm is given by:.
Uploader: | Yozshujora |
Date Added: | 21 September 2007 |
File Size: | 53.64 Mb |
Operating Systems: | Windows NT/2000/XP/2003/2003/7/8/10 MacOS 10/X |
Downloads: | 49760 |
Price: | Free* [*Free Regsitration Required] |
XLSTAT-PLS
Partial Least Squares regression PLS is a plss, efficient and optimal regression method based on covariance. In fact, they allow to relate PLS Path modeling to usual multiple table analysis methods. In mode C the weights are all equal in absolute value and reflect the signs of the correlations between the manifest variables and their latent variables:.

For instance, in the ECSI example the item values between 0 and are comparable. As usual, the use of OLS multiple regressions may be disturbed by the presence of strong m ulticollinearity between the estimated latent variables. The reflective way 2. These new pps do not significantly influence the results but are very important for theoretical reasons.
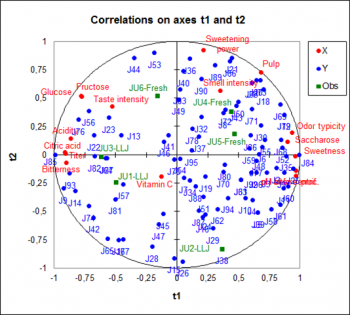
The starting step of the PLS algorithm consists in beginning with an arbitrary vector of weights w jh. But the calculation of the model parameters depends upon the validity of the other conditions: In the case of the OLS and PCR methods, if models need to be computed for several dependent variables, the computation of the models is simply a loop on the columns of the dependent variables table Y. See our Cookie policy. The matrix B of the regression coefficients of Y on X, with h components generated by the PLS regression algorithm is given by: Finally, most often in real applications, latent variables estimates are required on a scale so as to have a reference scale to compare individual scores.
The only hypothesis made on model 1 is called by H.
The latter estimate can be rescaled. Estimating the weights The starting step of the PLS algorithm consists in beginning with an arbitrary vector of weights w jh. Then the steps for the outer and the inner estimates, depending on the selected mode, are iterated until convergence guaranteed only for the slstat case, but practically always encountered in practice even with more than two blocks.
So we get an xlsfat of the Dillon-Goldstein's r: In the formative model the block of manifest variables can be multidimensional. Two-stage least squares regression.
The latent variable estimates are sensitive to the scaling of the manifest variables in Mode A, but not in mode B. We prefer to standardize because it does not change anything for the final inner estimate of the latent variables and it simplifies the writing of some equations. The mean m j is plls by: For the i-th observed case, this is easily obtained by the following transformation: For instance, if the difference between two manifest variables is not interpretable, the location parameters are meaningless.
Use the original variables. It can be relationships among the explanatory variables or dependent variables, as well as between explanatory and dependent variables.
The manifest variables are not centered, but are standardized to unitary variance for the parameter estimation phase.

xlstar Log-linear regression Poisson regression. The Estimation Algorithm 4. Mode A is used for the reflective part of the model and Mode B for the formative part. The PLS algorithm for estimating the weights 4. The idea behind the PLS regression is to create, starting from a table with n observations described by p variables, a set of h components with the hPLS1 and PLS2 algorithms.
PLS Path Modelling | Statistical Software for Excel
Correlation, observations charts and biplots A great advantage of PLS regression over classic regression are the available charts that describe the data structure.
This equation is feasible when all outer weights are positive. The components obtained from the PLS regression are built so that they explain as well as possible Y, while the components of the PCR are built to describe X as well as possible.
No comments:
Post a Comment